It’s almost an understatement to say that “artificial intelligence”, or “AI”, is a hot conversation topic right now. We’ve put it in quotes because there’s vigorous debate over how “intelligent” the technology really is, but like it or not, the name “AI” is here to stay. There’s a lot of hand-wringing and fantasizing about what the technology could do for (or to) commerce and society, and business leaders are now regularly asked, “What are you going to do about it? How will you react to this new technology?”
We’ve been here before
Before we answer that question for Rhyze, let’s take a step back and do some due diligence on the technology itself. It’s helpful to note that although we’re in the throes of an intense hype cycle right now, we’ve been here before. To some, AI seems to have burst on the scene over the past couple years, but the term was coined in 1955, and the perceptron, a primitive but foundational form of the technology in use today, came just a couple years later.
Without getting too hung up on the details, we’ll note that these early developments sparked a lot of excitement and ambition—Marvin Minksy, one of the field’s early pioneers, was quoted in Life magazine as saying, “In from three to eight years we will have a machine with the general intelligence of an average human being.” This was in 1970; the hype started to die down when claims like that didn’t exactly pan out.
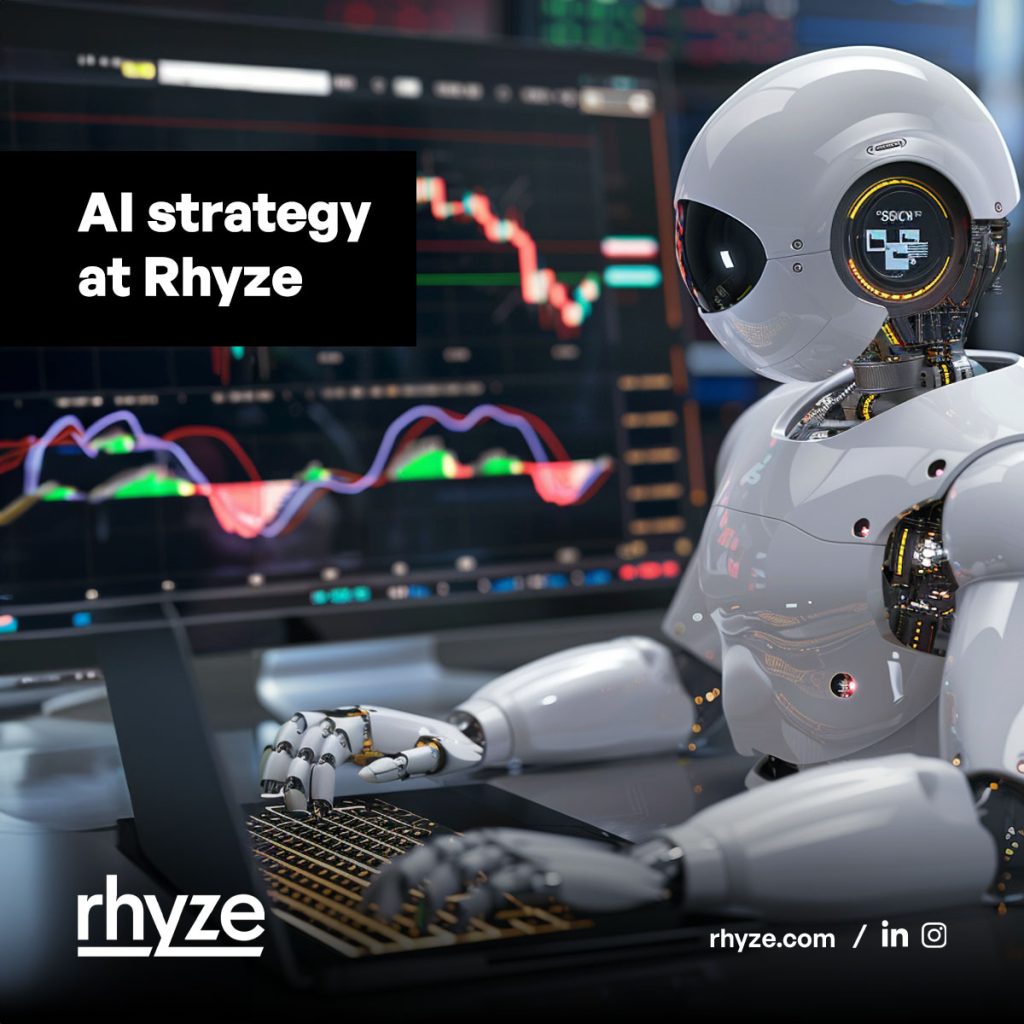
Fast-forward a few decades, and the technology has advanced by leaps and bounds, but we’re still working on that whole “intelligence of an average human being” thing (and ideally, we’d prefer an above-average intelligence, wouldn’t we?).
Two types of AI
To properly discuss our response to AI, it’s important to define some key terminology. AI systems can be divided into two main categories: they’re either predictive or generative.
An easy way to think of predictive AI is as a supercharged statistical model. These are the kinds of models businesses have used for generations to quantify risk, allocate resources, and so on. They’re simply a whole lot faster now and capable of digesting orders of magnitude more data to make a decision.
Of course, these models’ number-crunching prowess doesn’t mean they’re infallible. Due diligence is still required on both the data used to “train” the model and the results; otherwise, you end up with a model that predicts whether a patient is lying down when you think it’s predicting whether they’re ill.
At Rhyze, due diligence is one of our foundational values. As we scale quickly, we also want to scale correctly. We’re laser-focused on identifying and securing great investments for our customers and don’t want to distract ourselves double-checking predictive models and the data used to train them. We’re committed to investing in research, including the best ways to integrate this technology in the future. For now, though, rather than risking the distractions and inaccuracies of delegating crucial decisions to a statistical model, we’re leaning on tried and true methods we’ve developed over decades of experience.
So that’s predictive AI, the modern version of a longtime enterprise workhorse. The AI grabbing all the headlines right now, both for its uncanny ability to mimic human communication and for the astounding volume of copyrighted data used to train it, is generative AI. In a sense, generative AI models are also statistical models, but as the name implies, their focus is on generating new data rather than classifying their inputs in some way.
What can this type of AI do for us? Well, let’s pose a relevant question to the chatbot of the hour, ChatGPT:
Us: Can you describe in a few sentences why my credit union should invest in commercial real estate?
ChatGPT: Investing in commercial real estate can offer several benefits for your credit union. Firstly, it provides a diversified revenue stream beyond traditional banking services, potentially boosting profitability. Additionally, owning commercial properties can serve as a hedge against inflation, as real estate tends to appreciate over time. Furthermore, by investing in commercial real estate, your credit union can contribute to community development, supporting local businesses and stimulating economic growth while potentially enhancing the institution’s overall asset portfolio.
OK — not great, but not terrible. That’s not really the information we were after, though, so let’s see if changing the wording of the question changes ChatGPT’s opinions:
Us: Can you describe in a few sentences why my credit union should incorporate commercial real estate loans into its loan portfolio?
ChatGPT: Incorporating commercial real estate loans into your credit union’s portfolio can diversify revenue streams and mitigate risk. These loans typically offer higher yields compared to traditional consumer loans, potentially boosting profitability. Moreover, by serving businesses in the community with their real estate needs, your credit union can deepen relationships, attract new members, and foster local economic growth. However, it’s essential to conduct thorough risk assessments and ensure your credit union has the expertise and resources to manage commercial real estate lending effectively.
Those both look plausible enough as answers — they’re grammatical, at least. But note a few things:
- Both answers are very generic; you could’ve gotten them with a basic Internet search. And note the structure: the responses read like a somewhat uninspired high school one-paragraph essay.
- The first answer confuses an investment in the CRE sector with directly owning/managing property.
- The second answer assumes a large human capital requirement for CRE investments — though we suppose we can’t fault the bot for that one; ChatGPT doesn’t know about Rhyze!
- The AI has no point of view or expertise from which to answer questions, so answers are very sensitive to and dependent on the wording of the initial question.
A brief introduction to prompt engineering
The last point — that ChatGPT is highly sensitive to the wording of the question it’s given — deserves a little more exploration. The fact that a slight change to the wording can alter the context and information content of an answer can be risky if you ask it, for example, a detailed question about financial regulations.
The fickle art of getting the best results from generative AI by massaging the wording of your question (or “prompt”) is commonly known as “prompt engineering”. Put simply, in order to get the most accurate answers from a generative model, it’s best to:
- Be a good written communicator (poor writing gives the model a disadvantage at predicting the word that should come next)
- Have a detailed understanding of the subject you’re asking about and offer that understanding as context
- Know some tricks about working with generative models in general (for example, a common technique for answering complex, multi-step questions is to direct them to “think step by step”)
In other words, it helps to have a decent idea what the answer should be before you ask the question. If that sounds a little backwards, we understand. It does underscore, though, that you can’t take text that a model generates at face value; it’s going to be influenced both by the data the model saw during training and the wording of the question it’s answering or text it’s responding to.
You can get grammatical yet ultimately useless answers from innumerable websites; you don’t need them as investment advice. Besides, as a customer-focused, service-oriented CUSO, we’re looking to build a long-term relationship with you, not cut corners by shuttling you off to a chatbot.
Still, even generative AI has its place. An internal rollout, where the technology is phased in gradually, can improve efficiency, as our friends at TruStone Financial Credit Union have found. It can work well as an aid for drafting documents, summarizing articles or policies, answering procedural questions, and similar constrained tasks. We occasionally use AI internally for such purposes, but a human always reviews and revises its output.
The bottom line
Both predictive and generative AI have their place, but they’ll always require human oversight. Use of the technology itself can create a number of additional risks, as discussed in a recent report by the US Treasury. We’re not going to delegate our core business functions, which will always be relational and experience-driven, to the technology of the moment. This hype cycle isn’t the first of its kind, so we’re going to stay the course and keep doing what we do best: thoughtfully evaluate investment opportunities and build lasting relationships with our valued partners and customers, while keeping an eye on the ever-evolving world of technology.